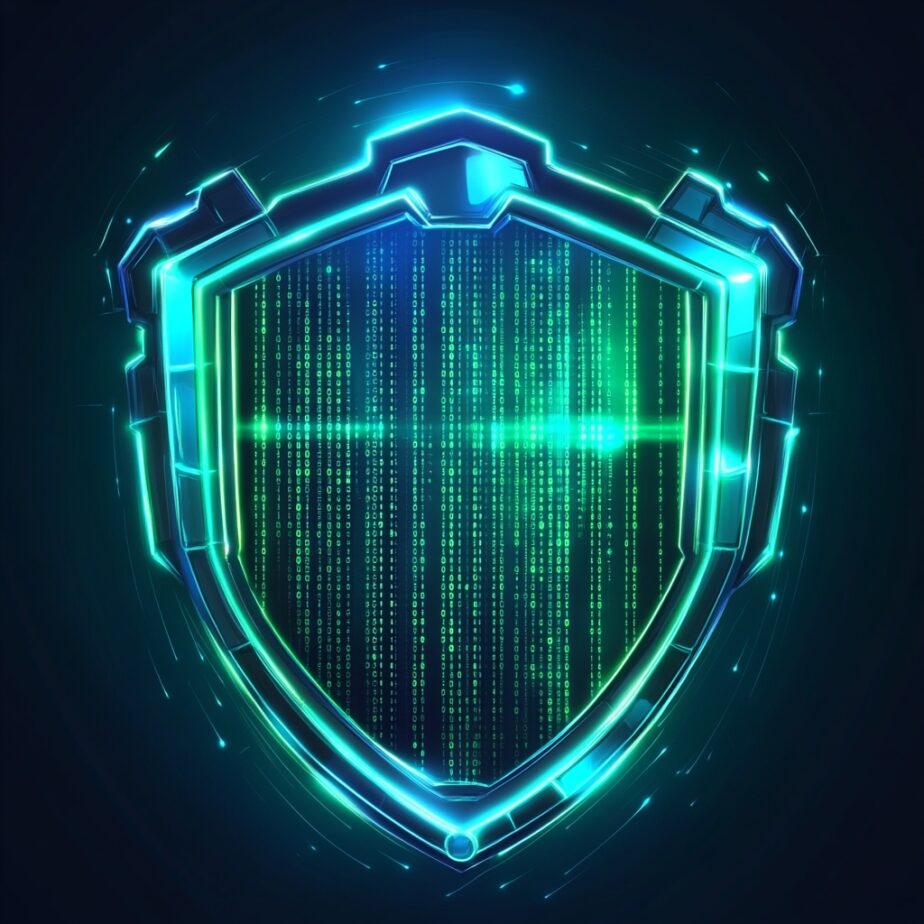
Introduction
The evolution of technology has brought both benefits and challenges, especially in the field of cybersecurity. The increasing number of devices connected to the internet, combined with the sophistication of cyber attacks, has become an increasingly difficult issue to manage. Traditional security solutions, such as firewalls and antivirus software, are struggling to keep up with
the growing complexity of attacks. In this context, the use of Machine Learning (ML) for threat and anomaly detection has emerged as an effective and promising approach.
The Challenge of Threat Detection in Modern Environments
In recent years, cyber threats have become more sophisticated and difficult to detect. Targeted attacks, Advanced Persistent Threats (APTs), and polymorphic malware are examples of vectors that challenge traditional approaches. These traditional methods often rely on lists of signatures or known attack patterns. While effective for previously identified threats, these methods can fail to detect new types of attacks, such as those exploiting zero-day vulnerabilities or insider threats that can disguise themselves as legitimate activities.
In this scenario, Machine Learning has emerged as a powerful solution. Unlike signature-based methods, ML can identify subtle patterns in large volumes of data and detect anomalous activity, even when it doesn't fit previously known behaviors.
This provides a much more robust security layer to deal with new or unknown threats.
How Machine Learning Works in Threat Detection
Machine Learning is a branch of artificial intelligence that allows systems to learn from data and make decisions based on detected patterns. To detect threats....